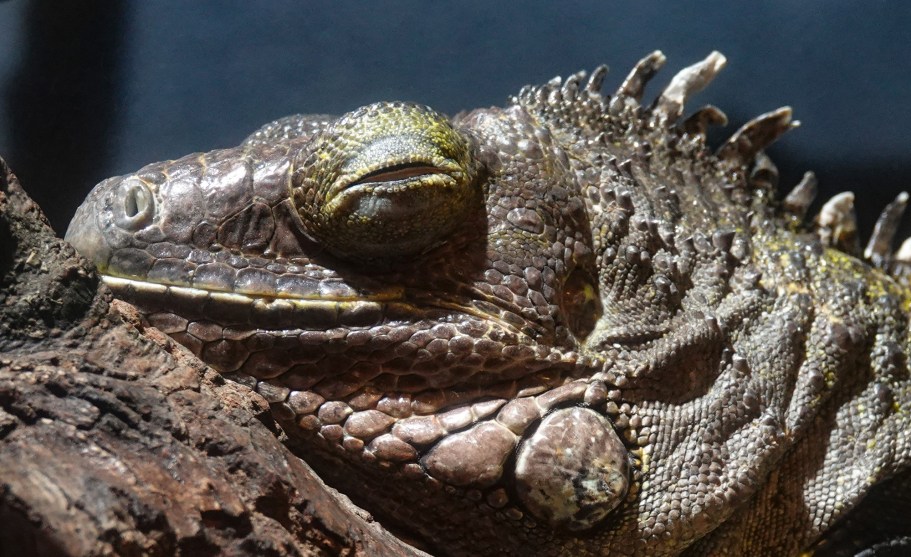
Over the past few years Amazon has been experimenting with new software to help them make better hiring decisions:
Automation has been key to Amazon’s e-commerce dominance, be it inside warehouses or driving pricing decisions. The company’s experimental hiring tool used artificial intelligence to give job candidates scores ranging from one to five stars — much like shoppers rate products on Amazon, some of the people said.
Hmmm. I’m not sure that machine learning is yet at a stage where it can really help much with this. On the other hand, it can be useful for ferreting out existing hiring patterns to see what Amazon’s managers seem to value most. So what did they find?
By 2015, the company realized its new system was not rating candidates for software developer jobs and other technical posts in a gender-neutral way. That is because Amazon’s computer models were trained to vet applicants by observing patterns in resumes submitted to the company over a 10-year period. Most came from men, a reflection of male dominance across the tech industry.
In effect, Amazon’s system taught itself that male candidates were preferable. It penalized resumes that included the word “women’s,” as in “women’s chess club captain.” And it downgraded graduates of two all-women’s colleges, according to people familiar with the matter. They did not specify the names of the schools….The Seattle company ultimately disbanded the team by the start of last year because executives lost hope for the project, according to the people, who spoke on condition of anonymity.
This is yet another confirmation—as if we needed one—that even the best-intentioned of us are bursting with internalized biases. Most of Amazon’s managers probably had no idea they were doing this and would have sworn on a stack of C++ manuals that they were absolutely gender neutral in their hiring decisions. In fact, I’ll bet most of them thought that they bent over backward to give female candidates a break. But down in the lizard part of their brains, it was the same old story as always: they preferred hiring men to women.
There’s a limit to how much you can take away from this. It’s another example of how implicit biases can affect us all, and a warning that any system we’re responsible for training—whether it’s fellow humans or digital computers—will pick up those biases. We all know we need to be careful about passing along our biases to the next generation, and it turns out we have to be equally careful about passing them along to the software we build.